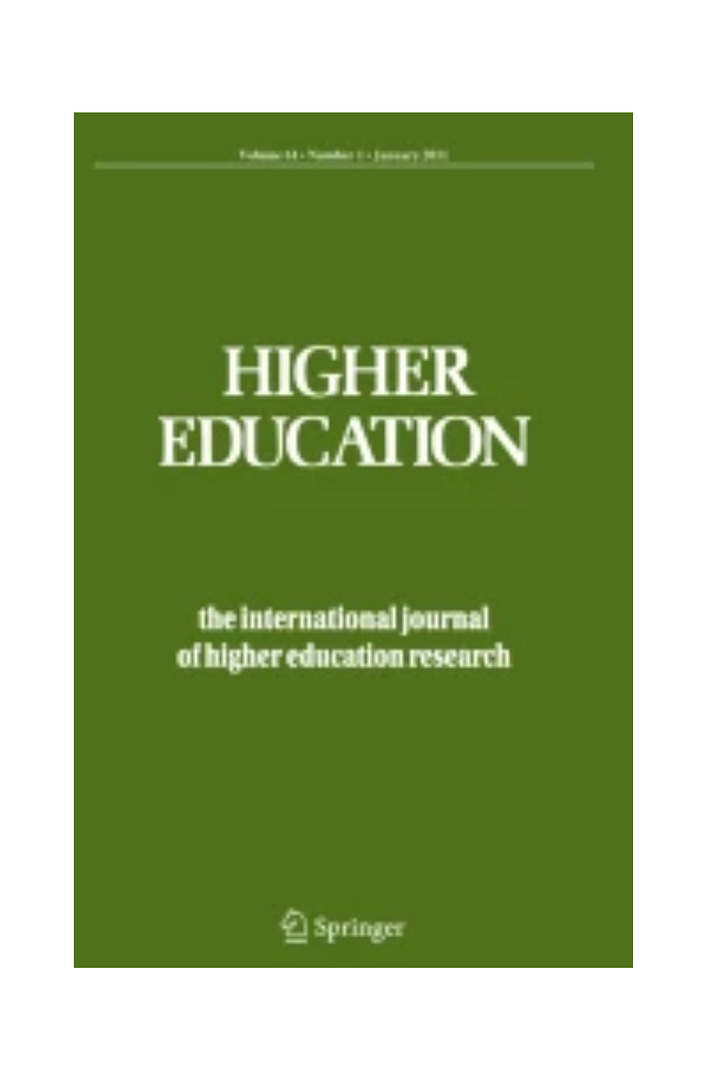
Jang-Tucci, K., Hora, M.T. & Zhang, J. Gatekeeping at work: a multi-dimensional analysis of student, institutional, and employer characteristics associated with unpaid internships. High Educ (2024). https://doi.org/10.1007/s10734-024-01254-6
Abstract:
Internships are recognized globally as a high-impact practice that substantially enhances students’ future prospects. However, concerns persist about their legality and potentially exclusionary nature. While prior research indicates participation varies based on key variables, such as gender and major, empirical work remains limited and tends to focus on univariate or single-actor explanations. We employ multi-actor models from management studies to analyze survey data (n=1153) from 13 U.S. institutions, nine of which are minority-serving institutions (MSI). The data reveal that only 30.3% of the students participated in internships, of which 43.4% were unpaid. Linear probability analysis results indicate that contrary to expectations, individual demographic characteristics, such as gender, were not signifcant predictors of internship compensation on their own. Instead, academic,
institutional, and employer characteristics emerged as signifcant predictors. Interaction analysis results highlight the combined efects of race, gender, major, MSI status, and employer characteristics in predicting participation in unpaid internships. Further, the data suggest that gender efects are largely infuenced by academic major afliation, emphasizing that unpaid internships do not uniformly afect all students but are predominant in specifc sub-groups. We conclude by proposing a strategy to eliminate unpaid internships in the interest of transformative social justice work.
Keywords: Work-based learning · Access · Unpaid internships · Minority-serving institutions · Inequality · Student success · Multi-actor frameworks · Linear probability model